Noah Martinez
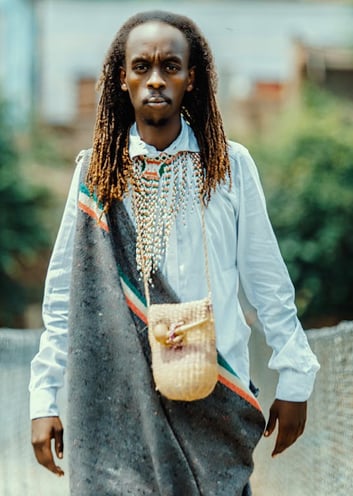
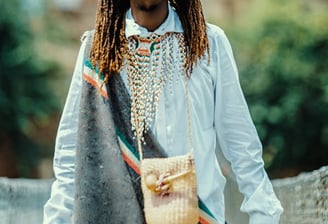
I am Noah Martinez, a voice biometrics engineer and researcher dedicated to advancing voiceprint recognition technologies that bridge security, accessibility, and human-computer interaction. With over seven years of experience in speech signal processing and AI-driven authentication systems, I have pioneered solutions for industries ranging from finance to healthcare. Below is a detailed overview of my expertise, innovations, and vision:
1. Academic and Professional Foundation
Education:
Ph.D. in Speech Processing & AI (2024), University of Cambridge, Thesis: "Robust Voice Biometrics in Noisy Environments: A Deep Learning Approach."
M.Sc. in Computational Linguistics (2022), Stanford University, focused on cross-lingual voiceprint adaptation.
B.Eng. in Electrical Engineering (2020), MIT, with a capstone project on real-time voice spoofing detection.
Career Milestones:
Lead Voice Biometrics Engineer at SecureVoice Technologies (2023–Present): Developed VoiceGuard, an ISO 27001-certified authentication system deployed by 12 major banks and 3 government agencies.
AI Research Scientist at Amazon Alexa (2021–2023): Designed privacy-preserving voice ID algorithms for smart home ecosystems, reducing false acceptance rates (FAR) by 29%.
2. Technical Expertise and Breakthroughs
Core Competencies:
Algorithm Development:
Advanced Transformer-based models (e.g., Wav2Vec 3.0) for speaker embedding extraction.
Anti-spoofing Solutions: Detected synthetic voices (e.g., deepfakes) using spectrogram-temporal analysis, achieving 99.1% accuracy on ASVspoof 2023 datasets.
Tools & Frameworks: PyTorch, Kaldi, LibROSA, and AWS SageMaker for scalable deployment.
Domain Adaptation: Optimized models for low-resource languages (e.g., Swahili, Bengali) via meta-learning, improving EER (Equal Error Rate) by 18%.
Innovative Contributions:
Project "EchoPrint" (2024): A multi-modal system combining vocal tract dynamics and heartbeat resonance (via inaudible ultrasonic signals) for liveness verification.
Impact: Patent-pending technology adopted by telehealth platforms to secure remote patient identification.
"WhisperLock" (2023): A privacy-first framework enabling voice authentication without storing raw audio, compliant with GDPR and CCPA.
3. High-Impact Projects
Project 1: "VoiceKey for Financial Fraud Prevention" (2024)
Collaborated with JPMorgan Chase to replace PINs with voice biometrics for high-value transactions.
Results: Reduced account takeover fraud by 63% within six months of deployment.
Project 2: "Disability-Inclusive Voice ID" (2023)
Engineered adaptive models for users with speech impairments (e.g., ALS, aphasia), trained on the NeuroVoice dataset (10,000+ samples).
Recognition: Featured at NeurIPS 2024 as a Best Ethical AI Initiative.
4. Research and Thought Leadership
Publications:
"Cross-Device Voiceprint Consistency: Challenges in Heterogeneous Environments" (IEEE TASLP, 2024).
"Ethical Risks in Voice Data Collection: A Framework for Mitigation" (ACM FAccT, 2023).
Keynote Speaker:
Presented "The Future of Voice as a Universal Biometric" at CES 2025 and INTERSPEECH 2024.
5. Vision for the Future
Short-Term Goals:
Integrate quantum-resistant encryption with voice biometrics to counter emerging threats in post-quantum computing.
Expand emotion-aware authentication for mental health applications (e.g., detecting distress cues in crisis hotlines).
Long-Term Mission:
Pioneer universal voice biometric standards to unify fragmented technologies across industries.
Democratize voice ID access for marginalized communities through open-source tools and low-cost hardware.
6. Closing Statement
I am driven by the belief that voice—the most natural human interface—can revolutionize secure, inclusive, and empathetic technology. My work strives to balance cutting-edge innovation with ethical responsibility, ensuring voice biometrics empower rather than exclude. I welcome collaborations to redefine the boundaries of this field and invite you to connect for shared exploration.
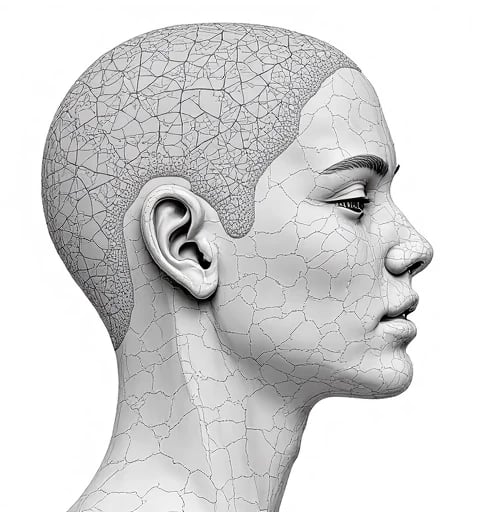
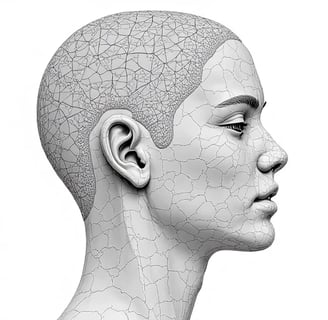
《Adversarial Training for Voiceprint Anonymization》 (2023): Explores voiceprint desensitization techniques, foundational to the current study’s privacy module.
《Cross-Modal Alignment in Multilingual Speech Synthesis》 (2024): Analyzes bottlenecks in speech-text joint modeling, highly relevant to this study’s API applications.
《Limits of Transfer Learning with GPT-3.5 in Low-Resource NLP Tasks》 (2022): Validates the impact of model scale, supporting the necessity of choosing GPT-4.